Table Of Content
- Learning protein fitness models from evolutionary and assay-labeled data
- Optimization algorithms without guarantees
- Computational Redesign of Metalloenzymes for Catalyzing New Reactions
- Extended Data Fig. 8 Targeted unconditional and fold-conditioned protein binder design.
- Functional-motif scaffolding
- Protein design articles from across Nature Portfolio
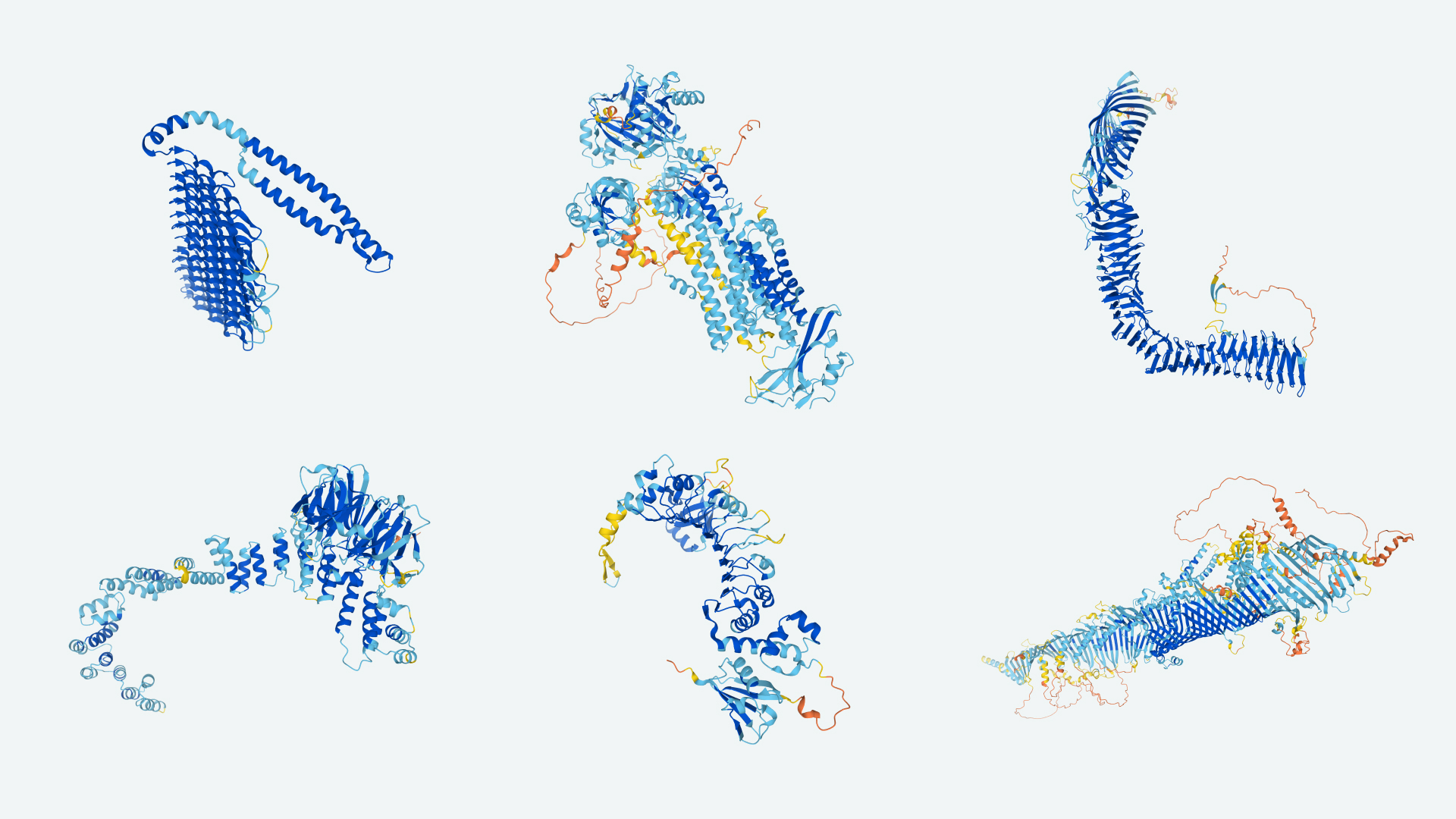
Designs such as HE0902 (and future similar large assemblies) should be useful as new nanomaterials and vaccine scaffolds, with robust assembly and (in the case of HE0902) the outward facing N and C termini offering many possibilities for antigen display. Grigoryan et al. implemented a set of rule selections to assemble a superstructure of peptides that coat single-walled nanotubes (SWNTs).29 They matched the periodicity of an α-helix to the periodic pattern surface of a SWNT via Ala Cβ methyl contacts to form a supercoil of α-helical coiled coils. In the presence of mixed types of SWNTs, the designed peptides preferentially sequestered the targeted nanotube species to produce stable aqueous suspensions. Rational protein design techniques must be able to discriminate sequences that will be stable under the target fold from those that would prefer other low-energy competing states. Thus, protein design requires accurate energy functions that can rank and score sequences by how well they fold to the target structure.
Reshaping protein design with function-first, AI-guided engineering - Phys.org
Reshaping protein design with function-first, AI-guided engineering.
Posted: Mon, 20 Nov 2023 08:00:00 GMT [source]
Learning protein fitness models from evolutionary and assay-labeled data
In the following, we highlight recent developments in scoring functions for membrane proteins and for interactions with nonprotein molecules, as well as scoring approaches that learn from structures in the PDB. There are many areas in the field of the computational de novo protein design where significant progress is needed. To make large sequence optimization problems computationally tractable, scoring functions use a number of approximations such as implicit solvation models and pairwise decomposable energy terms. Current backbone geometry sampling methods are limited to certain secondary structures and fold topologies.
Optimization algorithms without guarantees
However, designing switches could be seen as a more tractable problem because the external trigger can introduce a large free-energy bias toward one state, making the design success less sensitive to scoring errors. An early study described a protein designed to switch between two distinct target folds triggered by the addition of Zn2+ (154). The authors used a Monte Carlo side-chain design method to optimize the sum of energies of the two folded states, showing that it is possible to design protein switches by solving a single-objective optimization problem. Following similar principles, other proteins were designed to change the oligomerization state in response to a pH change (155) (Fig. 6A) or change conformations in the presence of Ca2+ (156) (Fig. 6B). A modular protein switch that senses a small molecule was designed through an induced dimerization mechanism (12) (Fig. 6C). A ligand binding site for farnesyl pyrophosphate was designed de novo at the interface of a protein–protein heterodimer complex.
Computational Redesign of Metalloenzymes for Catalyzing New Reactions
C, side chain design methods that exploit backbone flexibility outperform fixed backbone methods (98). E, neural networks can predict the probabilities of sequences given a backbone structure (102, 103) (red). Generative machine learning models design sequences by latent space sampling (104, 105, 106, 107, 108) (green). The TR-Rosetta neural network predicts the probability of the structure of a given sequence.
Designing hydrogels with the DeForest Lab
Notably, the structure prediction neural network from TR-Rosetta (42) can be repurposed for sequence optimization (109). For a protein sequence, the TR-Rosetta neural network predicts distances, angles, and dihedrals for every pair of residues. A loss function is defined as the difference between the prediction and the target structure. The gradient of the loss is then back-propagated through the TR-Rosetta neural network to optimize the sequence.

Divalent transition metal ions show distinct preferences for specific coordination geometries (for example, square planar, tetrahedral and octahedral) with ion-specific optimal sidechain–metal bond lengths. RFdiffusion provides a general route to building up symmetric protein assemblies around such sites, with the symmetry of the assembly matching the symmetry of the coordination geometry. We designed C4 protein assemblies with four central histidine imidazoles arranged in an ideal Ni2+-binding site with square-planar coordination geometry (Fig. 5b). Diverse designs starting from distinct C4-symmetric histidine square-planar sites had good in silico success with the histidine residues in near ideal geometries for coordinating metal in the AF2-predicted structures (Supplementary Fig. 9).
Scoring interactions with nonprotein molecules
A similar method was also used to identify mutations that increase brightness and shift excitation peaks,64 allow GFP to fold faster,65 and introduce a number of additional properties useful for a wide range of applications. Comparison of force-field performance in simulations of the 78 amino acid protein, ubiquitin. Each column corresponds to a given force field (as indicted) and each row corresponds to a different model for explicit solvent (as indicated).
Functional-motif scaffolding
We construct a RF-based diffusion model, RFdiffusion, using the RF frame representation that comprises a Cα coordinate and N-Cα-C rigid orientation for each residue. We generate training inputs by noising structures sampled from the Protein Data Bank (PDB) for up to 200 steps22. For residue orientations, we use Brownian motion on the manifold of rotation matrices (building on refs. 23,24). To enable RFdiffusion to learn to reverse each step of the noising process, we train the model by minimizing a mean-squared error (m.s.e.) loss between frame predictions and the true protein structure (without alignment), averaged across all residues (Supplementary Methods). This loss drives denoising trajectories to match the data distribution at each timestep and hence to converge on structures of designable protein backbones (Extended Data Fig. 2a). The m.s.e. contrasts to the loss used in RF structure prediction training (frame aligned point error or FAPE) in that, unlike FAPE, m.s.e. loss is not invariant to the global reference frame and therefore promotes continuity of the global coordinate frame between timesteps (Supplementary Methods).
Here, we use computational protein design to create novel miniproteins that bind to human TLR3 with nanomolar affinities. Cryo-EM structures of two minibinders in complex with TLR3 reveal that they bind the target as designed, although one partially unfolds due to steric competition with a nearby N-linked glycan. Multimeric forms of both minibinders induce NF-κB signaling in TLR3-expressing cell lines, demonstrating that they may have therapeutically relevant biological activity. Our work provides a foundation for the development of specific, stable, and easy-to-formulate protein-based agonists of TLRs and other pattern recognition receptors. A 4-fold symmetric TIM barrel was designed using the blueprint fragment assembly strategy described above (34). Experimental characterization of the designs revealed important hydrogen bonds defining the strand register between repeat units.
Expanding the types of molecules supported by scoring functions is critical for designing such protein functions. Scoring functions for DNA (127) and RNA (128) have been successfully applied to structure prediction and design (129, 130). Recently, a scoring function was developed for saccharide and glycoconjugate structures (131) (Fig. 4B). Benchmarking results on docking problems showed that the scoring function has the ability to predict binding of glycan ligands. Small molecules have highly diverse combinations of chemical groups, making it challenging to transfer parameters calculated for representative molecules to other molecules. A new approach (132) simultaneously optimized all parameters in a small-molecule energy function guided by thousands of small-molecule crystal structures.
Toward new functions, recent computational advances have led to the ability to generate precise geometric variations in de novo–designed protein families, mimicking the ability of evolution to precisely tune the shapes of the members of protein families for new activities (28, 32). Although these designed proteins are not close in sequence to any naturally occurring proteins, principles from structures in the PDB are still the guiding design. Such principles are useful for generating new protein structures through assembly from continuous (33, 35) or discontinuous (25, 36, 37) three-dimensional elements, as well as for the development (38) and optimization (39, 40) of design energy functions used to rank design candidates. Moreover, the most recent developments of deep learning for protein structure prediction (41, 42, 43) foreshadow new methods in the design, taking advantage of learned principles of the protein structure (44, 45). For binder design from target structural information alone, previous work required testing tens of thousands of sequences12.
No comments:
Post a Comment